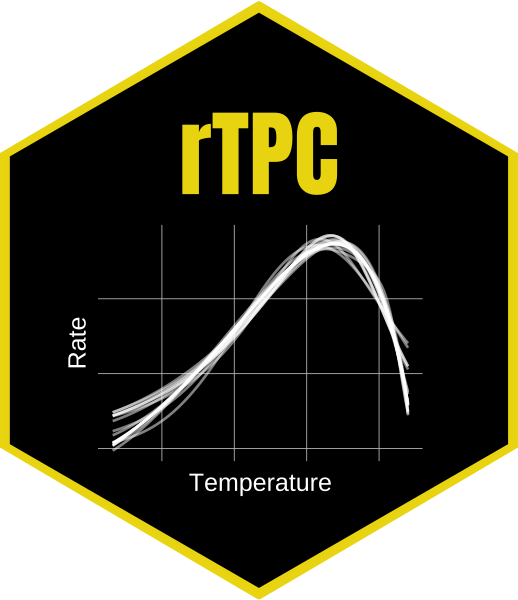
Analytis-Kontodimas model for fitting thermal performance curves
Source:R/analytiskontodimas_2004.R
analytiskontodimas_2004.Rd
Analytis-Kontodimas model for fitting thermal performance curves
Value
a numeric vector of rate values based on the temperatures and parameter values provided to the function
Details
Equation: $$rate = a \cdot \left(T - T_{\text{min}}\right)^2 \cdot \left(T_{\text{max}} - T\right)$$
Start values in get_start_vals
are derived from the data or sensible values from the literature.
Limits in get_lower_lims
and get_upper_lims
are based on extreme values that are unlikely to occur in ecological settings.
References
Kontodimas, D. C., Eliopoulos, P. A., Stathas, G. J. & Economou, L. P. Comparative temperature-dependent development of Nephus includens (Kirsch) and Nephus bisignatus (Boheman) (Coleoptera: Coccinellidae) preying on Planococcus citri (Risso) (Homoptera: Pseudococcidae): evaluation of a linear and various nonlinear models using specific criteria. Environ. Entomol. 33, 1–11 (2004).
Examples
# load in ggplot
library(ggplot2)
# subset for the first TPC curve
data('chlorella_tpc')
d <- subset(chlorella_tpc, curve_id == 1)
# get start values and fit model
start_vals <- get_start_vals(d$temp, d$rate, model_name = 'analytiskontodimas_2004')
# fit model
mod <- nls.multstart::nls_multstart(rate~analytiskontodimas_2004(temp = temp, a, tmin, tmax),
data = d,
iter = 200,
start_lower = start_vals - 10,
start_upper = start_vals + 10,
lower = get_lower_lims(d$temp, d$rate, model_name = 'analytiskontodimas_2004'),
upper = get_upper_lims(d$temp, d$rate, model_name = 'analytiskontodimas_2004'),
supp_errors = 'Y',
convergence_count = FALSE)
# look at model fit
summary(mod)
#>
#> Formula: rate ~ analytiskontodimas_2004(temp = temp, a, tmin, tmax)
#>
#> Parameters:
#> Estimate Std. Error t value Pr(>|t|)
#> a 2.249e-04 7.640e-05 2.944 0.016381 *
#> tmin 1.438e+01 2.763e+00 5.203 0.000562 ***
#> tmax 4.900e+01 9.767e-01 50.170 2.49e-12 ***
#> ---
#> Signif. codes: 0 ‘***’ 0.001 ‘**’ 0.01 ‘*’ 0.05 ‘.’ 0.1 ‘ ’ 1
#>
#> Residual standard error: 0.3081 on 9 degrees of freedom
#>
#> Number of iterations to convergence: 45
#> Achieved convergence tolerance: 1.49e-08
#>
# get predictions
preds <- data.frame(temp = seq(min(d$temp), max(d$temp), length.out = 100))
preds <- broom::augment(mod, newdata = preds)
# plot
ggplot(preds) +
geom_point(aes(temp, rate), d) +
geom_line(aes(temp, .fitted), col = 'blue') +
theme_bw()